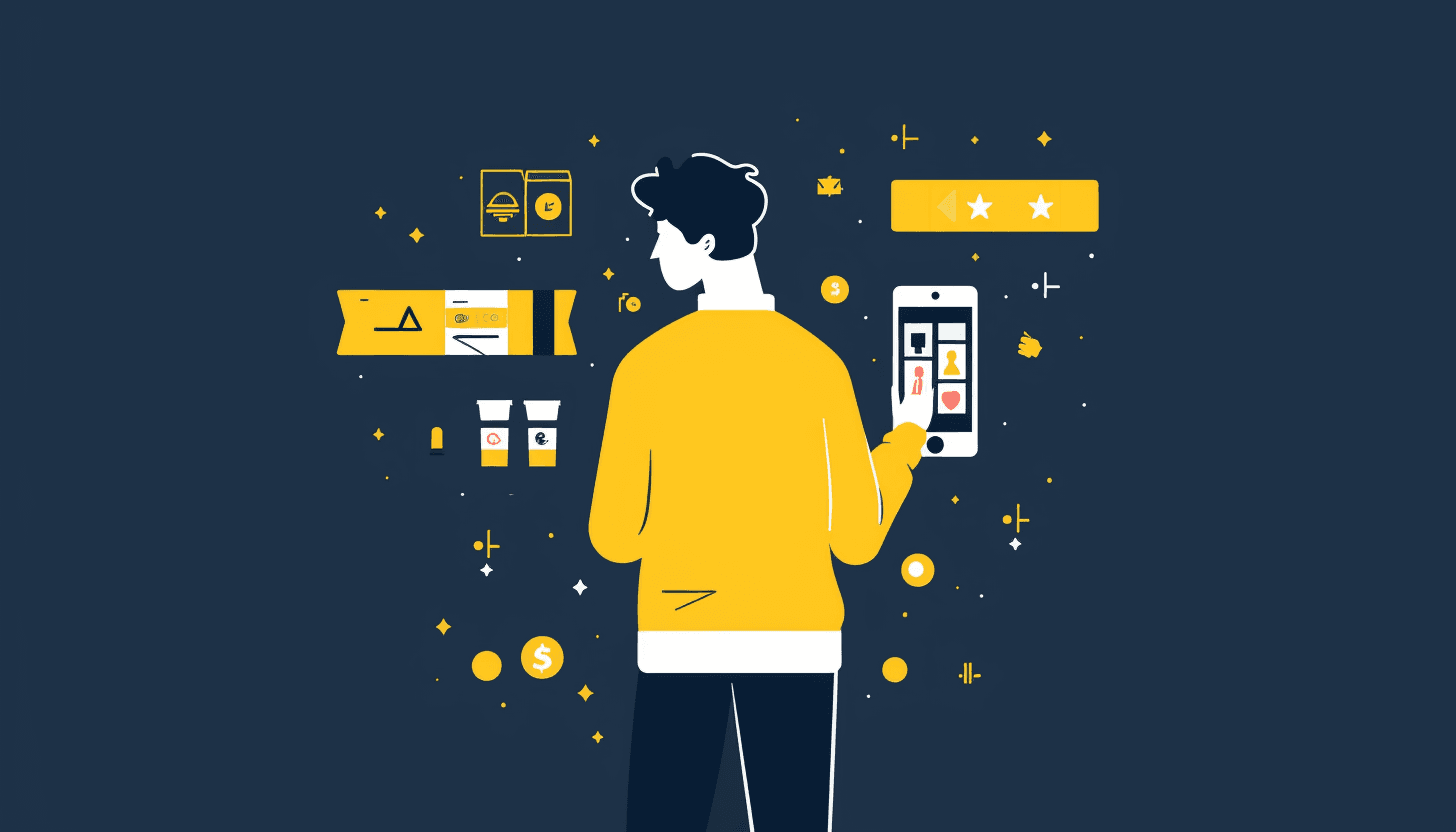
Recommendation system
Industry
Retail
Team setup
1 developer
Timeline
2 months (ongoing)
Problem to solve
Offering the right product at the right time is crucial in the retail industry. On rieker-eshop.hu there are 5000+ available shoes, making it really hard for customers to find the product that they are actually looking for. Showing only the products that fit the taste of the customer saves time and effort for them, and increases the overall customer satisfaction.
Challenge
To understand a given customer’s needs - and suggest just the right products - we can build a recommendation system, using machine learning (ML). We need a solution that doesn’t require extra ML expertise, can be built by our existing team, scales automatically, secure and cost-effective.
Outcome
We built a recommendation system with a serverless-first approach. We implemented a scalable solution to collect user interactions - this gives us the base dataset for the ML training jobs. Then we utilized an off-shelf solution from AWS to train and deploy models. Finally, we integrated this with our existing rieker-eshop.hu website.
Recommendation system
Industry
Retail
Team setup
1 developer
Timeline
2 months (ongoing)
Problem to solve
Offering the right product at the right time is crucial in the retail industry. On rieker-eshop.hu there are 5000+ available shoes, making it really hard for customers to find the product that they are actually looking for. Showing only the products that fit the taste of the customer saves time and effort for them, and increases the overall customer satisfaction.
Challenge
To understand a given customer’s needs - and suggest just the right products - we can build a recommendation system, using machine learning (ML). We need a solution that doesn’t require extra ML expertise, can be built by our existing team, scales automatically, secure and cost-effective.
Outcome
We built a recommendation system with a serverless-first approach. We implemented a scalable solution to collect user interactions - this gives us the base dataset for the ML training jobs. Then we utilized an off-shelf solution from AWS to train and deploy models. Finally, we integrated this with our existing rieker-eshop.hu website.
Recommendation system
Industry
Retail
Team setup
1 developer
Timeline
2 months (ongoing)
Problem to solve
Offering the right product at the right time is crucial in the retail industry. On rieker-eshop.hu there are 5000+ available shoes, making it really hard for customers to find the product that they are actually looking for. Showing only the products that fit the taste of the customer saves time and effort for them, and increases the overall customer satisfaction.
Challenge
To understand a given customer’s needs - and suggest just the right products - we can build a recommendation system, using machine learning (ML). We need a solution that doesn’t require extra ML expertise, can be built by our existing team, scales automatically, secure and cost-effective.
Outcome
We built a recommendation system with a serverless-first approach. We implemented a scalable solution to collect user interactions - this gives us the base dataset for the ML training jobs. Then we utilized an off-shelf solution from AWS to train and deploy models. Finally, we integrated this with our existing rieker-eshop.hu website.
Recommendation system
Industry
Retail
Team setup
1 developer
Timeline
2 months (ongoing)
Problem to solve
Offering the right product at the right time is crucial in the retail industry. On rieker-eshop.hu there are 5000+ available shoes, making it really hard for customers to find the product that they are actually looking for. Showing only the products that fit the taste of the customer saves time and effort for them, and increases the overall customer satisfaction.
Challenge
To understand a given customer’s needs - and suggest just the right products - we can build a recommendation system, using machine learning (ML). We need a solution that doesn’t require extra ML expertise, can be built by our existing team, scales automatically, secure and cost-effective.
Outcome
We built a recommendation system with a serverless-first approach. We implemented a scalable solution to collect user interactions - this gives us the base dataset for the ML training jobs. Then we utilized an off-shelf solution from AWS to train and deploy models. Finally, we integrated this with our existing rieker-eshop.hu website.
Browse more of our successes
Find more of our successes and learn about our processes!
Browse more of our successes
Find more of our successes and learn about our processes!
Browse more of our successes
Find more of our successes and learn about our processes!
Browse more of our successes
Find more of our successes and learn about our processes!